Robotics: The Era of AI and Autonomy
The expected valuation of the smart global robot industry is poised to grow by $44.44 billion during 2021-2025, according to ResearchandMarkets.com. NASDAQ even has a CTA Artificial Intelligence and Robotics Index designed to track enablers, engagers or enhancers in the artificial intelligence or robotics sectors. The smart robot market is growing and industries including aerospace, defense, medical, manufacturing, freight and others are all looking at ways to leverage robots to improve their services and products. However, Artificial Intelligence impacts everything on a macro level.
Key drivers for using AI in robotics applications
As humans, a fundamental driver for the use of AI in robotics is to improve our own conditions. We’re constantly looking for a better phone, TV, car, or smart appliance to improve our quality of life. Sometimes, we look at ways to improve our physical environment with innovations like wind power and solar panels. Other times we are looking for ways to reduce our exposure to dangerous jobs or we simply want to find ways to avoid dull and repetitive tasks.
Industries recognize the human desire to improve our quality of life. They respond by innovating, creating new technologies and optimizing their processes. These innovations, however, drive the market to continue to innovate and create the next newer, better, faster AI solution.
Challenges presented by the emerging AI-enabled robotics
This constant cycle of innovation presents some challenges. IDC predicts that the amount of data in the world will grow 10 times by 2025 to 163 zettabytes, up from 16.1 zettabytes of data generated in 2016. Data feeds artificial intelligence. Today, robots are generating a lot of data, primarily from the sensory input needed to operate. A vastly higher level of machine awareness makes for an industrial environment rich in sensor-derived data but potentially stretched too thin in the processing and analytics departments. Traditional computing strategies and frameworks can be overwhelmed.
A Solution at the Edge
Pushing all of this data out somewhere else for processing — into the cloud — is no longer practical, nor does it make sense. We increase productivity, because with artificial intelligence and access to so much data, the robot can make decisions much faster than humans — and statistically speaking, the robot will always make the best possible decision.
With so many machines, sensors, and data in the mix, computing will have to take place increasingly on the edge. The robots themselves will be better equipped to perform more activities and make more decisions autonomously.
A robot driven by data gathered and processed at the edge could detect the likelihood of its own breakdown or, at the very least, the failure to maintain quality standards. Communicating with the other robots on the assembly line, the at-risk machine shuts down while others adapt their workflow in real time to make up for the missing worker. The production line slows but doesn’t stop. A human technician intervenes, making the needed adjustment or repair, and then the system returns to full speed. The only way this and related capabilities can be realized is through the edge.
Security binds data and connectivity
As robots become mobile, collaborative, edge resident, and connected to internal and external sensors and IoT devices, the data-rich ecosystem opens itself to multiple access points for would-be hackers. Companies may find themselves vulnerable to malware, cyber ransom, production delays, and business disruption. What’s more, cyberattacks targeting highly nimble, powerful robotic systems also come with some serious physical safety concerns.
Security should not be an afterthought. Some basic actions to take include enabling secure boot, more effectively managing deployed software using containers technology, leveraging concepts such as time partitioning to minimize the potential for a denial of service (DoS) attack, or better segregate software components with mandatory access control (MAC).
Systems integrators also need to understand the machines they’re installing and the overall environment, with an eye toward identifying potential access points and hardening vulnerable targets. Finally, the operator’s IT team needs to be actively engaged, monitoring threats, and updating security measures.
What to look for in a real-time technology stack
If 75% of all data will be consumed at the edge, then 25% of data will be pushed out. As data moves from point A to point B, we have to choose the right stack to conduct the transfer of information because all stacks are not created equal. Companies will want to adapt the protocol stack to the use case with interoperability in mind. TSN and OPC-UA are two examples worth considering.
Time sensitive networking (or TSN) is an evolution of what used to be called audio video bridging (AVB). TSN ensures that if you send data from point A to point B, the data will arrive within a certain timeframe and accuracy.
OPC-UA allows the user to have a vendor agnostic communication system for the purpose of having machines talk to other machines.
Conclusion
In order to help AI machines become more autonomous, all of the knowledge that can be acquired through the entire system will be necessary. The edge cloud is now an ecosystem of machines and provides the best connectivity for AI machines and data. The business implications of the new era of AI and Autonomy mean that the dynamics for decision-making in robotic systems are evolving rapidly. What we might once have seen as incremental steps now become opportunities for transformation.
Comments (0)
This post does not have any comments. Be the first to leave a comment below.
Featured Product
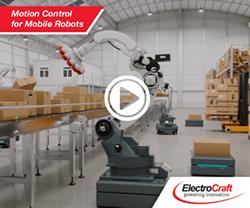