Deep learning is a machine learning technique that teaches computers to learn by example just as we learned as a child. We see this technology in autonomous vehicles.
Top Article from 2019 - What is Deep Learning
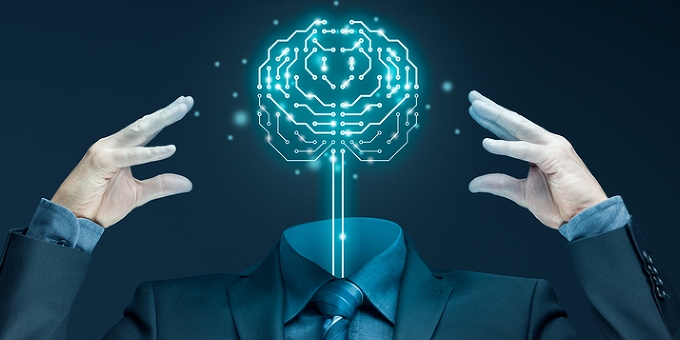
Len Calderone for | RoboticsTomorrow
Deep learning is capturing the attention of all of us as it is accomplishing outcomes that were not previously possible. Deep learning is a machine learning technique that teaches computers to learn by example just as we learned as a child. We see this technology in autonomous vehicles. It enables the vehicle to distinguish between different objects on the road and enables the vehicle to stop when it sees a red light. An autonomous vehicle can determine when it is safe to move forward or to remain stationary.
In deep learning, a computer becomes proficient at performing tasks from images, text, or sound, and can realize state-of-the-art accuracy, many times exceeding human implementation.
We often hear the terms: AI (artificial intelligence), machine learning and deep learning. So, what are the differences? All machine learning is AI, but not all AI is machine learning. AI is a general term for any computer program that does something smart. Deep learning is a subset of machine learning, and machine learning is a subset of AI.
Artificial intelligence is an area of computer science that stresses the creation of intelligent machines that work and respond like humans. The basic procedure of machine learning is to provide training data to a learning algorithm, which in turn generates a new set of rules, based on inferences from the data. By using different training data, the same learning algorithm could be used to produce diverse models. Deducing new instructions from data is the strong suit of machine learning. The more data that is available to train the algorithm, the more it learns.
When the term deep learning is used, it usually refers to deep artificial neural networks. Deep artificial neural networks are a set of algorithms that have set new bests in accuracy for critical problems, such as image recognition, sound perception, and language processing. Deep learning accomplishes perception accuracy at higher levels than ever before in areas such as consumer electronics, and it is vital for safety-critical applications such as autonomous vehicles. Current developments in deep learning have improved to the point where deep learning does better than humans in performing many tasks.
Inspired by the neurons that make up the human brain, neural networks comprise layers that are connected in adjacent layers to each other. The more layers there are, the deeper the network. A single neuron in the brain, receives as many as 100,000 signals from other neurons. When those other neurons fire, they apply either an exciting or inhibiting effect on the neurons to which they are connected. If the first neuron’s inputs add up to a certain base voltage, it will fire as well.
In an artificial neural network—just like the brain—signals travel between neurons. But instead of firing an electrical signal, a neural network allocates emphases to a variety of neurons. A neuron biased a great deal more than another neuron will wield more of an effect on the next layer of neurons. The final layer patches these weighted inputs together to come up with an answer.
These neural networks are made of layers of weighted neurons. Only they are not modelled on the workings of the brain. They are inspired by the visual system.
Every layer within a neural network utilizes a filter across the image to pick up explicit shapes or characteristics. The first few layers distinguish larger features, such as diagonal lines, while the following layers pick up finer details and organizes them into complex features.
Credit: Aphex34/Wikimedia Commons
Similar to an ordinary neural network, the final output layer is fully connected, which means that all of the neurons in that layer are connected to all neurons in the previous layer. The layers of neurons that are sandwiched between the first layer of neurons (input layer) and the last layer of neurons (output layer), are called hidden layers. This is where the neural network endeavors to solve problems. Reviewing the activities of the hidden layers can tell a lot about the information the network has learned to extract from the data.
Traditional neural networks only contain 2-3 hidden layers, while deep networks can have as many as 150. Large sets of labeled data are used to train deep learning models, using neural network architectures that learn features directly from the data without the need for manual feature extraction.
Deep learning machines don't require a human programmer. This is possible because of the extraordinary amount of data we collect and consume. Data is the power for deep-learning models. Because of this, deep learning machines are already being used for practical purposes.
As deep learning continues to mature, we can expect many businesses to use machine learning to enhance customer experience. There are already deep-learning models being used for chatbots and online self-service solutions.
Machine translation isn’t new, but deep learning is facilitating enhance automatic translation of text by using stacked networks of neural networks and allowing translations from images.
In the past, black and white movie images had to be hand colored, which was very time consuming and costly. Now this process can be automatically done with deep-learning models, which can automatically colorize grayscale images based on Convolutional Neural Networks, which features a fusion layer that allows an artist to merge local information dependent on small image areas with largescale prior images.
Advanced natural language processing and deep learning can help to filter news subjects in which you are interested. News aggregators using this new technology can filter news based on sentiment analysis, so you can create news streams that only cover the happening news containing stories of interest.
Another remarkable ability of deep learning is to identify an image and create an intelligible caption with proper sentence structure for that image just as though a human was writing the caption.
A deep learning machine can even generate text by learning the punctuation, grammar and style of a piece of text. It can use the model it created to automatically generate completely new text with the proper spelling, grammar and style of the example text. James Patterson—watch out.
The evolution of deep-learning machines is projected to pick up the pace and create even more innovative uses in the next few years. Deep-learning applications can educate a robot just by observing the actions of a human carrying out a task or use the connection from several other AIs in order to perform an action. A human brain processes input from past experiences. A deep learning robot will execute tasks based on the input of many different AI opinions.
The content & opinions in this article are the author’s and do not necessarily represent the views of RoboticsTomorrow
Comments (0)
This post does not have any comments. Be the first to leave a comment below.
Featured Product
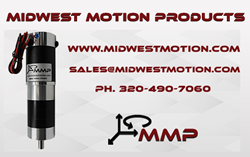