Combining on-vehicle sensor data with high resolution maps adds another safety level to the autonomous driving system. The automotive industry is looking to its partners and suppliers as they develop HD maps.
Autonomous Driving Systems
Interview with Bibhrajit Halder for | AutoSens 2016
Reprinted with permission - AutoSens 2016
With a growing stable of world-class designers, product developers and technologists enticed from a diverse range of industries and seemingly unrelated disciplines, the California-based start-up enjoys a growing reputation for uninhibited innovation alongside a playful love of mystery.
In a rare interview with a current staffer working on autonomous driving systems, we caught with Dr Bibhrajit Halder, a Software Technical Specialist in the ADAS and Self-Driving team, who is currently preparing to speak at the AutoSens conference, which will be held at AutoWorld, Brussels, between 20-22 September 2016.
Hi Bibhrajit, what’s your background?
I have been working on autonomous robotics since attending graduate school at Vanderbilt University, Tennessee, where I worked on supervisory control system of autonomous robots.
The central theme of supervisory control is to detect any anomaly or fault in the robot itself. Clearly understand how severe the fault at its current situation to make a decision that is both safe and graceful for robot operation and performance.
In the context of vehicle perception, monitoring the vehicle itself (which I called Vehicle Health Manager) is one of the important components of perception among three. The other two crucial components are Localization, i.e., position of the vehicle related to map or other known reference, and Environment Model, i.e., understanding what is around the vehicle to determine what path planning is safe and efficient.
In my professional career, I worked on Caterpillar Autonomous Mining Truck for over 6 years, where we developed all three components as I just describe above, then moved to Ford, where I continued my work on localization given limited and lower accuracy sensor information.
Currently, I manage a team of engineers to develop complete perception solution from the ground-up at an automotive startup called, you guessed it: Faraday Future.
What are the most important lessons you learned developing autonomous vehicles at Caterpillar?
At Caterpillar we developed Level 4 autonomous mining trucks that have been running in production for over 2 years now. There are over 50 autonomous mining trucks that are running at various mining around the world. These autonomous mining trucks operate 24/7 with scheduled 8 or 12 hour intervals [for routine inspections, refuels, etc].
The Caterpillar solution, the Command for Hauling 793F truck has an enviable safety record, with a vehicle up to 390 metric tonnes (860,000 lb) the safety principles applied to such a dangerous operation as open cast mining are a great learning ground for the unpredictability and decision-making that autonomous vehicles will have to face.
Caterpillar has been doing research work on this for more than 10 years but the project started with a blank page.
The biggest takeaway for me is that making a reliable, safe, and robust autonomous vehicle is extremely difficult. Making it work 90-95% of the time takes a lot of effort; however, the last 5% takes more than twice the time and energy of first 95%. We continued resolving issues deep into our beta testing at the mine, while gathering enormous amount of data. You have to go through detail testing and analyzing huge amount of data to understand all the unusual ‘fringe’ cases to build safe and reliable autonomous vehicle.
For example, during testing if the autonomous vehicle is close to violating the expected behavior:
If the vehicle is getting too close to the lane boundary (whereas the vehicle should to stay in the middle of the lane), then we did a manual intervention and took the control back. We’d also collect and play back the data where we let the vehicle run without manual intervention to understand what it would have done if we didn’t take over and also why it did that.
Google has produced a vast amount of data from autonomous vehicles [largely because the company have accrued so many driverless miles]. Analyzing and post processing that data is central to the development of any self-driving vehicle.
Fault detection and isolation were important aspects of autonomous vehicles at Caterpillar – How does that translate to a passenger vehicle?
The Supervisory Control of autonomous vehicle includes three main components:
- detecting system anomaly or faults;
- understanding the significance of that fault at current situation, and finally;
- making decisions that are safe and graceful for the vehicle.
These map directly into passenger vehicles.
I think about this as what we do while driving: Imagine driving on highway and you notice that the tyre pressure is low. We slow down and look for an exit and take the vehicle to the nearest gas station, where we check the tyre. Here as a driver, we detected the fault, made a decision what to do, and took the safest action. In the autonomous vehicle, a Supervisory Control Manager replaces this functionality.
[This example would be supported by the Tyre Pressure Management System, a legal requirement for remote monitoring in many countries since 2012.]
How does Faraday Future’s approach change the way you think about developing your own autonomous vehicles?
Without going into much detail, as a start-up we enjoy the need to push the boundaries of self-driving vehicle both in terms of functionalities and time to the market, while making safety and reliability the utmost importance. Our advantage is that we are starting with a clean paper and there is no legacy to carry.
In the United States, the National Highway Traffic Safety Administration (NHTSA) has proposed a formal classification system:
Level 0: The driver completely controls the vehicle at all times.
Level 1: Individual vehicle controls are automated, such as electronic stability control or automatic braking.
Level 2: At least two controls can be automated in unison, such as adaptive cruise control in combination with lane keeping.
Level 3: The driver can fully cede control of all safety-critical functions in certain conditions. The vehicle senses when conditions require the driver to retake control and provides a "sufficiently comfortable transition time" for the driver to do so.
Level 4: The vehicle performs all safety-critical functions for the entire trip, with the driver not expected to control the vehicle at any time. As this vehicle would control all functions from start to stop, including all parking functions, it includes unoccupied vehicles.
As we look towards autonomous driving, what do you see as the biggest challenges to sensor technology?
The industry is rapidly moving towards Level 3 and 4 autonomous vehicles (see box), so LiDAR will become a much more important sensor along with the mix of radar and camera technology. We are still on the lookout for a LiDAR solution that provides high resolution data at a competitive price point, where other OEMs are hunting for solutions at or below $100 to reach production volume. When you add the total amount of all the sensors involved to make a car Level 3 or 4 autonomous capable, you see the costs involved add up very quickly.
The industry sees high definition mapping as an important part of autonomy at Level 3 and beyond. Combining on-vehicle sensor data with high resolution maps adds another safety level to the autonomous driving system. The automotive industry is looking to its partners and suppliers as they develop HD maps.
One of the big questions being discussed today is distributed vs centralized processing, what is your view on that?
The answer is not one versus another. In nearly any current production vehicle there are over 100 ECUs in the vehicle. Currently, there are individual ECMs for each specific ADAS features. As the industry moves further to Level 3 and 4 autonomy, there would be one centralized processing unit, which would be more powerful than anything in the production vehicle today, for example NVIDIA recently announced DrivePX2.
Sensors will also become smarter and will process lower level information. For example, in a radar sensor, it makes sense to be doing FFT (Fast Fourier Transform) and other signal analysis inside the radar chip, but classification of an object should be done in the central processing unit as it is desirable to combine other sensor information in the classification process.
LiDAR is emerging as an important technology for perception, especially as lower cost options become available. However, there are potentially interference issues with more widespread use. Do you think this will impact uptake of LiDAR?
Yes, the industry is looking at signal interface issue as the use of LiDAR increases. But I don’t think this will be the limiting factor for adopting LiDAR technology in the vehicle.
The issues everybody is concerned with are cost, size, time to market, and reliability. Handling signal interface can be solved in the software and technically this is well understood problem.
Finally, we are very pleased to have you join the AutoSens community, what are you hoping to gain from your participation?
Engaging with the community and learning from others are what I am excited about. You have done a wonderful job organizing this and I look forward to join the community.
The content & opinions in this article are the author’s and do not necessarily represent the views of RoboticsTomorrow
Comments (0)
This post does not have any comments. Be the first to leave a comment below.
Featured Product
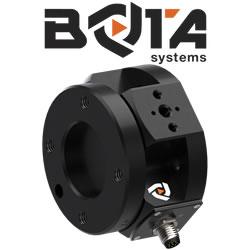